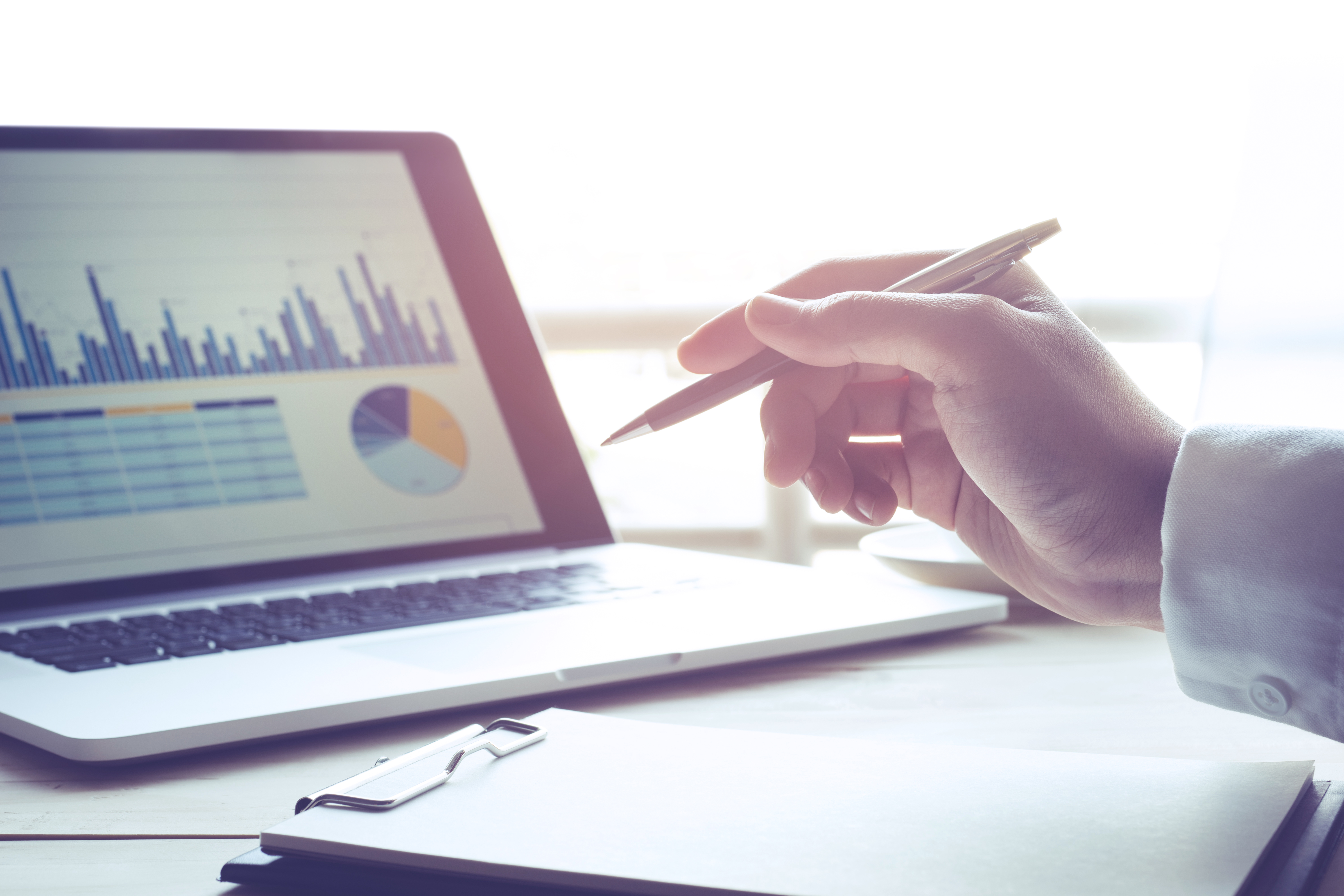
The term ‘Data Analytics’ is becoming more and more commonly used…
Data analytics are also the end game of the BI System Builders’ Cornerstone Solutions®. But what are data analytics? This article describes the term as used by the BI System Builders. While experts in other organisations may have a different definition this paper will serve to make the BI System Builders’ thinking clear. We will start by considering the ‘classic’ data analytics first becoming popularised pre-millenium and then move on to the new breed data analytics made possible through new technologies.
Firstly, it’s important to note that the term data analytics is frequently used interchangeably with other names such as analytical applications, business analytics, and as a component of Performance Management Applications to name but a few. BI System Builders only use one terminology, that of ‘data analytics’. That said, data analytics tend to fall into three categories, business analytics, statistical analytics, and predictive analytics. In practice these analytic types may be combined.
Now, all organisations capture data. This may be through sales invoices, captured in a call centre, via a website, delivery notes from suppliers, research questionnaires, financial transactions, point of sale transactions or in the area of scientific research or engineering and so on and so forth. The data captured is in a raw format and will usually be disjointed across several of your data capture and transaction systems. This data can be very difficult to interpret so an activity may be undertaken to bring the data into some form of structured database tables even if it’s just a one to one mapping. You may hear people referring to these database tables as ‘landing tables’, ‘staging tables’, or ‘Operational Data Store (ODS) tables’. ODS tables may be ‘raw’ in form or may be highly structured though a data modelling technique known as entity modelling or third normal form (3NF or BCNF) modelling. Either way all these table types can be very complex to ask questions against. At this stage data viewed in reports is often referred to as operational reporting. The data in operational reports is not summary but low level, detailed, granular, disjointed, and may include codes that don’t seem to make any sense. Have you ever tried to read through and make sense of a million rows of limited format technical data and codes in a day? That detail of data may have its use for certain operational reporting purposes but for business user purposes it needs to be more readable and needs to be presented at a higher level being consolidated or summarised in some way to make it readable.
The transaction/source system/raw data captured has been increasing in high volumes over the years. This raw data may be structured or unstructured data and can also be highly volatile in nature. Furthermore the exponential growth of the internet, mobile devices, social media, scientific research, and technology has meant the potential for enormous amounts of detailed personal information to be captured everyday – your web clicks, your buying behaviour, your communications, your location, GPS co-ordinates, your posts, your reviews, etc., etc., hence the entrance of GDPR in 2018.
Historically, business intelligence tools have been evolved to extract, clean and format the raw data then join it up together turning it into valuable and useful business information presented through data analytics. The business intelligence tools are relevant and highly valuable when used in conjunction with a structured data warehouse. The data is often summarised and may also be pre-calculated in other ways as well. The information can then be used to discover insights into your business or organisation. The reports are sometimes shared across the organisational enterprise and can be based on enterprise wide data in an enterprise data warehouse (EDW), hence the term, enterprise reporting.
Let’s take a look at the classic data analytic capability in the data warehouse and then consider data analytics in the new big data era. Data analytics go further than simple reporting by adding extra insights/intelligence into the reports. This is done in several ways. Some of the classic ways are the use of dynamic parameters for on the fly analysis such as period on period, ‘drill down drill up’ through hierarchical data structures, a drill across capability linking business process areas across the business, predictive engines, and ‘what if’ analysis that allow the user to play out different scenarios by changing the values of variables in the data analytic.
Dynamic parameters allow the user to refresh the data in the data analytic and change the question being asked by selecting contextual values in a prompt. For example with period on period analysis you may start by viewing sales today compared with sales yesterday. The user can then easily change the data analytic to compare current month to date sales versus last month to date sales or last year’s month to date sales etc. The simple data analytic below is probably cleverer that it looks as it includes several calculated fields and all the analysis periods can be changed on the fly. When they are changed the other dependent fields automatically recalculate. The data analytic also has a hierarchical data structure drill down capability and dynamically changing sentences which automatically capture values such as the name of the city with the highest percentage change.
Building on the concept of drill down and drill up, this is a technique used to navigate through a hierarchical structure in your data. For example you may capture sales data by location. In an data analytic with a hierarchical data structure you may view sales for all locations and then with a click of the mouse ‘drill down’ to sales by region, and then drill down again to sales by city and down to individual sales outlets. This can all be achieved within a single data analytic.
The drill across capability allows you to navigate through a series of linked data analytics. These are often based on a logical work process flow. The linkage is achieved through code written behind the scenes and invisible to the user. Data analytics can be clever enough to know the context of the information you are viewing such as location, time period and product and pass this context to the next data analytic.
Predictive and ‘what if’ capabilities allow the user to play out different scenarios. For example you could measure the impact of inflation. To do this a percentage value would be input into a dynamic parameter prompt. An algorithm in the data analytic would then read the value and recalculate itself to show you the impact. Other algorithms may be designed that are complex in nature and may use statistical analysis techniques such as regression and correlation.
There are many other things that you can achieve with data analytics such as cycle time analysis. The picture below is a cycle time chart. In this case the chart is analysing the customer order actual cycle time but the technique can be applied to any business process cycle time. The information has great value. If you can measure the duration of individual stages within a business process you can identify those that are least efficient. By then addressing any inefficiency within a stage you can make it more efficient, reducing its duration and consequently cost, thus improving the profitability of the process.
It is also common to measure things such as Key Performance Indicators (KPIs), customer churn, customer life time value, stock turn. It is possible to develop time series analysis, strategy maps, balanced scorecards, and six sigma based statistical process charts across business process areas, not just one process area in isolation. Data analytics based on a business intelligence system could technically be used to combine HR data with finance data, and finance data with supply chain data and so on and so forth. The bottom line is that data analytics will help you find efficiency and effectiveness in your business according to your needs. This type of data analytic is already used by many organisations although others still struggle to realise them. Other examples of data analytics are also accessible through social media platforms including Google Analytics, LinkedIn Analytics, and YouTube Analytics.
However, we no longer live in the slowly changing world of the data warehouse alone. The cost of technology is reducing, the 64 bit processor is here and large amounts of RAM can now be exploited. Furthermore, technological advancements have yielded in-memory applications such as SAP HANA and IBM’s DB2 Blu Acceleration and distributed architectures and file systems such as Apache Hadoop. The combination of these things means that enormous volumes of data can be ‘released’ from the systems in which they have been captured and processed fast – near real-time, and real-time fast. Things that were once beyond budget are now starting to come within budget.
Earlier I asked the question about have you ever tried to read through a million rows of data? I wasn’t joking, I’ve witnessed business users attempting to do this is in a report and then find the bits of information that they needed. Of course, they give up quite quickly and don’t get repeat opportunities because of the system degradation this type of report processing has historically caused. Now I’ll ask another question, “Have you ever tried to read though a hundred billion rows of data in a report?” Preposterous? – yes, impossible to process? – no, some organisations now capture data at the terabyte plus scale.
It is impossible to make meaning out of such high volume, highly volatile and disparate data as is now becoming available without new breed data analytics. But these data analytics do not need to be reactive, running against previously processed data, they are now being exploited in a pre-emptive way. Consequently the term ‘data scientist’ has been popularised and is frequently closely associated with predictive analytics (machine learning). Volumes of raw data may be so huge that they are referred to as a data lake. On these huge data lake volumes an algorithm or logic may be executed on the unstructured data in the form of programs as data is streamed into the data pipeline. Further programs may also include training models and deep neural networks which seek to find previously hidden relations within the data and make predictive outcomes. Historically, a related albeit more simple type of activity was referred to as data mining, now highly sophisticated versions of the activity may be associated with the names artificial intelligence (AI), machine learning (ML), and data science. Ultimately, they are a ‘pre-emptive strike’ functions applying weightings and algorithms to data label inputs and outputting a result set of probabilities. As sophisticated as today’s data scientists may be their algorithms, coding, and complex modelling, the concept of using decision engines, training models, predictive engines, data mining, business activity modelling (BAM), trending, time series analysis, correlation, regression, tests of significance, pattern detection,probabilities, disparate unstructured source mapping, and the identification homogeneous behaviour have their early roots set in the past. However, now the technology and the data is available these things will be exploited in data analytics for business and research like never before. For a clear example of this consider the idea ‘big data means marketing science’, but the topic of data collection, technological capabilities, privacy, and ethics deserves an article all of its own…
You must be logged in to post a comment.